논문에서 제시한 내용만 보고 구현한 코드 약간의 Naive한 점이 있지만 확실히 ResNet 등의 CNN 모델보다 학습속도가 빠르다! 그럼 바로 시작 1. Setup 기존과 동일하다. import torch.nn as nn import torch import torchvision import torchvision.transforms as transforms from torch.utils.data import DataLoader import torch.optim as optim import time import numpy as np import random import torch.backends.cudnn as cudnn seed = 2022 torch.manual_seed(seed) torch.cuda...
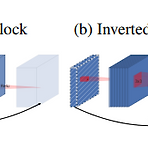
V1에서 달라진 점과 Architecture 중심으로 이야기를 하려고 한다. 0. Abstract MobileNet V2는 Bottleneck 간 skip-connection과 Inverted Residual Structure를 바탕으로 설계되었다. Block 안 expansion layer는 lightweight depthwise conv를 사용한다.(MobileNet v1과 같다.) 또한 representational power를 유지하려면 narrow layers에선 non-linearities를 제거하는 것이 중요하며 이를 실험적으로 확인했다. 이러한 아이디어를 바탕으로 실험한 결과 성능향상을 확인할 수 있었다. 1. Intro 현재 SOTA 모델들은 높은 computational cost(res..